Why IBM Is Untethering Watson AI Software from Its Cloud
AI models are best trained right where the data is, and most customer data isn’t in IBM’s cloud.
February 16, 2019
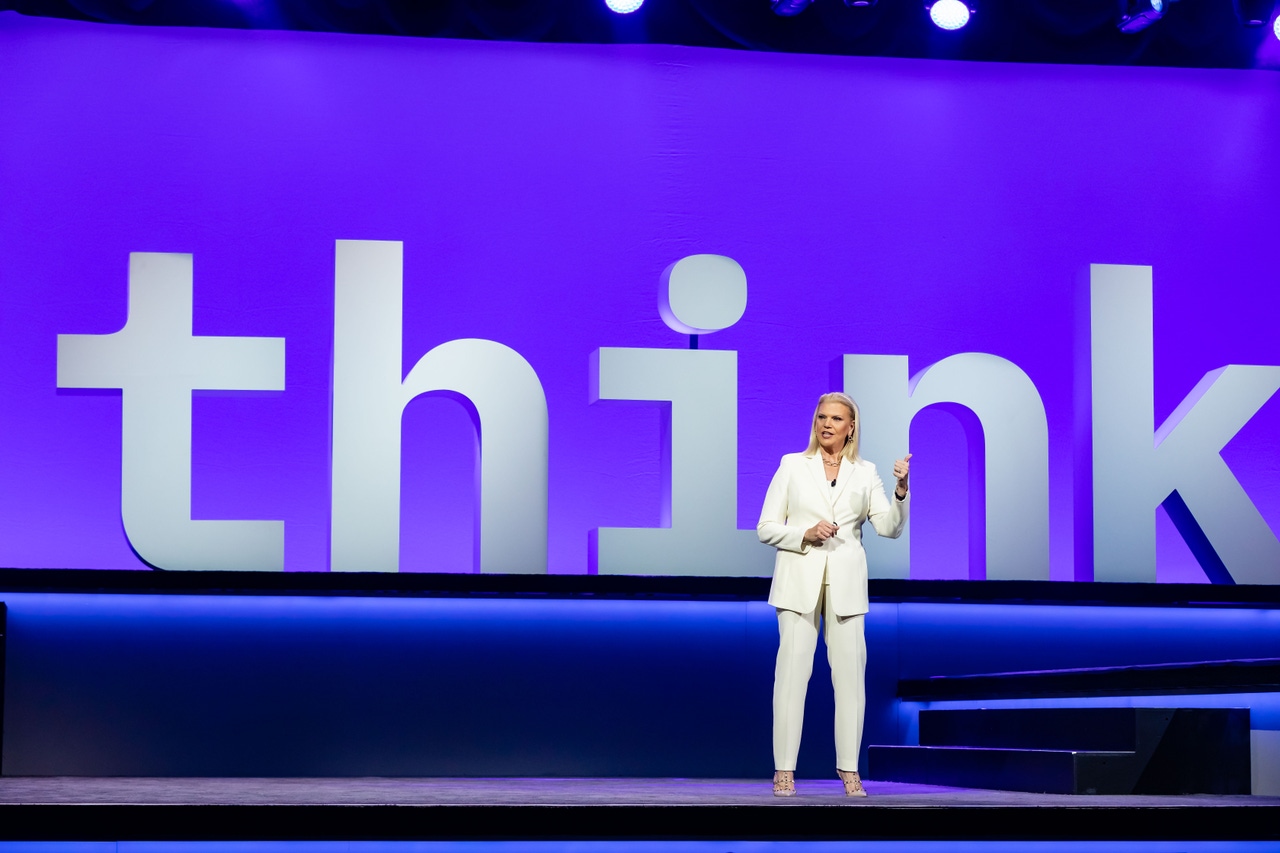
It’s cheaper and more practical for a company to run software that trains a machine learning model in the same location where its data lives. And most companies that store data in the cloud store it in Amazon Web Services or Microsoft Azure – the two market share leaders in raw cloud infrastructure services.
That’s one good reason for IBM to enable its suite of AI tools called Watson to run in the data centers of its biggest cloud rivals. But most of the data companies have isn’t stored in any public cloud, which is a good reason to let them run Watson in their own corporate data centers.
IBM is now doing both. At its Think conference in San Francisco this week, the company announced Watson Anywhere, making the software that until now has only been available on its own cloud infrastructure platform-agnostic.
“You need to do your training close to where your data is,” Daniel Hernandez, VP of IBM Data + AI, told Data Center Knowledge. In most cases today that means moving data to the same data center where your AI training engine is. “We’re not asking our customers to make that choice.”
Moving terabytes upon terabytes of enterprise data to the cloud isn’t simply a matter of provisioning a high-bandwidth network link to an AWS data center. The volume of data is so large, moving it over a Wide Area Network is impractical and too expensive. “You basically have to ship disks in order to move mission critical data” from one data center to another,” he said.
That’s why there are appliances like Azure Data Box, Google’s Transfer Appliance, and Amazon Snowball, which are basically big rugged storage devices cloud providers ship to you so you can load them with your data and ship them back for uploading to their clouds. IBM has one too: the IBM Cloud Mass Data Migration device.
When training a machine learning model, it’s not enough to move data once. The datasets have to be constantly refreshed and updated to continuously improve the model. This further makes storing a data set in one location and running a model in another impractical.
No Interest in ‘Commodity’ IaaS Business
IBM made Watson portable by building Kubernetes-based Watson microservices for IBM Cloud Private for Data, a cloud-native platform for collecting and managing enterprise data. Open source Kubernetes is what makes them compatible with other companies’ platforms. The microservices are for Watson OpenScale and Watson Assistant, a management console for AI projects and a tool for building conversational interfaces into applications, respectively.
The move highlights a shift in IBM’s cloud strategy away from raw infrastructure services – which as a result of price wars between Amazon, Microsoft, and Google has become a low-margin commodity business – and toward sophisticated software tools packaged for enterprise needs and delivered as cloud services.
Depending on which analyst you ask, IBM is a third, fourth, or fifth-largest cloud provider, its market share close to Google’s and Alibaba’s. But Google has been spending billions on cloud data center infrastructure every quarter (as have Amazon and Microsoft), while IBM has not. Since it’s no longer trying to compete with the hyperscale giants in Infrastructure-as-a-Service, its pace of data center investment isn’t likely to change.
IBM isn’t interested in being a “commodity” IaaS provider, Robin Hernandez, director for IBM Cloud Private and Multicloud Platform, told us. Its cloud strategy now is focused on modernizing its middleware portfolio and selling Watson and analytics services for enterprise use cases, leveraging public cloud to do that, she explained.
A Shot at Non-Cloud AI Workloads
Untethering Watson from IBM’s own cloud infrastructure also opens the opportunity for it to go after AI workloads companies don’t deploy in the cloud, which reportedly is a growing market. For example, signaling that there’s rising demand from companies for their own machine learning infrastructure, Nvidia recently launched a referral program to help those customers find colocation data centers that can support these often extremely power-dense computers.
While companies often start experimenting with machine learning models in the cloud, an Nvidia marketing director explained to us earlier, as their models mature and have to scale, they find that having their own AI infrastructure is more effective and economical. Watson Anywhere gives IBM a shot at this segment of the market.
Today, IBM can say its AI tools supporting any platform makes it stand out among cloud providers. But taken on its own this differentiation is likely to lose a lot of steam in the future. All the biggest cloud providers have hybrid cloud options in various stages of readiness, and it’s probably only a matter of time before customers can get GPU instances in an AWS Outposts rack in their own data centers; or on Azure Stack hardware (work on this appears to be already on the way); or on Cisco servers deployed as an on-premises extension of Google Cloud Platform.
If this is something customers truly want, IBM’s biggest rivals will eventually make their AI tools available in customers’ own data centers, once the hardware piece is there.
About the Author(s)
You May Also Like