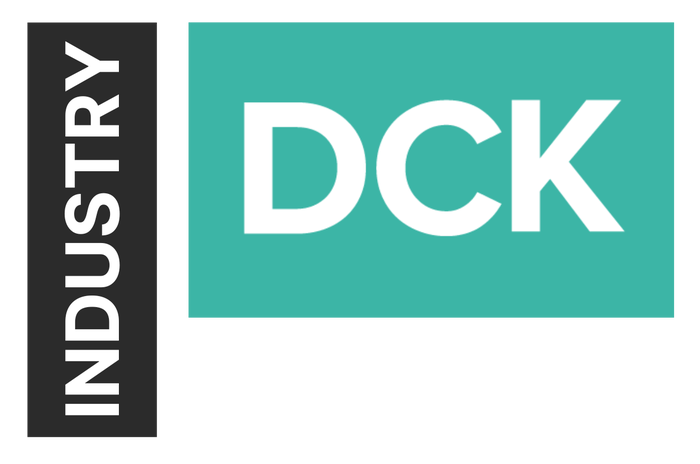
Insight and analysis on the data center space from industry thought leaders.
Big Data Remakes the Data Center
Despite cloud computing, many data centers are snake pits of complexity, writes Mike Wronski of StrataCloud. Here are four tenets that can help simplify data center operations, save money and improve performance and user experience.
October 14, 2014
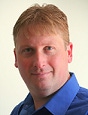
Mike Wronski is Vice President of Systems Engineering and Customer Success with StrataCloud
Your company’s been spending the past year or two investigating how the business and IT can support Big Data initiatives across key areas such as predictive demand, customer service, and R&D. But Big Data also has a big role to play in remaking your data center, too.
It’s high time for the data-driven data center. That may sound like an oxymoron, but the fact is, data centers need an overhaul.
Despite cloud computing, many data centers are snake pits of complexity. A survey by Symantec Corporation found that pervasive use of cloud computing, virtualization and mobile technologies may diminish investments in blade servers and other modernization technologies meant to simplify the data center.
Growth in data volumes and business applications combined with high user expectations for speed and uptime place added pressures on data center managers. Tackling these issues to make data centers more responsive and efficient begins with a more precise, data-driven approach to IT operations management, one founded in Big Data technologies and strategies.
These four tenets can help simplify operations, save money and improve performance and user experience for the business:
One: storage of real-time machine generated data
IT operations data is produced at increasingly high rates within the data center from all corners of the business and the Web. This real-time machine data includes performance data from components such as servers, storage and networking equipment as well as application response times, click to action, and load times.
Access to data from these different platform components is now easier thanks to modern APIs, virtualization, and software-defined infrastructure. Data center operators have ample opportunity to make use of these diverse data types through Big Data analytics tools and thereby gain powerful insight into operations.
A massive increase in raw operational data demands new technologies and strategies for storing it. Even though hard disk storage costs have declined over the years, those cost declines haven’t kept pace with the growth of data production that some IT organizations are experiencing.
Fortunately, Big Data platforms specializing in compression and deduplication are becoming more available to help with the cost and management challenge. A large portion of the data available is time-series performance data (metric, measurement, and timestamp). To obtain highly-accurate analytics, the original raw data must be stored for longer periods of time andreferenced frequently, making general purpose databases and storage schemes a poor choice for management.
To further reduce storage costs, IT organizations should choose a platform with a storage model that scales out horizontally across many smaller nodes. This will balance the query across nodes reducing response time and enabling intelligent analysis of the raw data.
Two: predictive modeling
Many companies count on accurate forecasting to better execute on business goals, and that advantage doesn’t stop with customer-facing business challenges. Predictive models are also important in the data center and may cover resource utilization, resource demand, failure, and problem prediction. These models surface possible issues before they become real problems and play a critical role in procurement and capacity planning.
However, providing good models means having sufficient data on which to base the models. Since storage of granular data is challenging, a shortcut is to water down the data into averages, instead of using the original raw data. Yet doing this usually results in predictions with a high margin of error.
To provide more relevant predictive modeling, the data behind the models must be collected frequently and from across the application stack. This enables IT organizations to accurately predict when applications will have issues and to optimize resources on the fly for both demand and cost.
Three: cross-stack visualization of business applications
IT organizations still typically operate in silos such as virtualization, compute, storage, networking and applications. While each organization generates plenty of usable data, often using their own preferred tools, real value comes from merging the data in context of the applications. Therefore, cross-stack visualization requires integrating data from all hardware and software involved in running the applications.
Consider the exercise of judging the capacity needs for adding 500 new virtual machines. Increases in storage, network, and CPU are needed but without correlating all of them together you may miss an important point: the storage layer also consumes network capacity so the network capacity must increase substantially more. Without cross-stack analytics giving the full picture, operations teams can wind up chasing contention problems at the network layer. If cross-stack visibility is available, it’s possible to quickly eliminate areas that are not the sources of problems. That usually results in faster time to resolution.
To get started on cross-stack visibility, encourage teams to share and store data centrally. Groups can continue to use their domain-specific management tools but allow those tools to push data into a central Big Data repository.
Four: distributed in-memory analytics
The value of real-time intelligence is clear, but getting there is not easy due to the volume of data streaming into the organization. Traditionally, IT has performed analysis in batch mode, yet that’s not viable with today’s virtualized data center where decisions need to be made at any point in time.
Distributed in-memory analytics entails keeping relevant portions of data in-memory and performing the analytics as new data arrives or is aged off. The concept is similar to distributed storage, and helps improve the efficiency and speed of analytics programs by splitting large tasks into subtasks for calculation, which can be combined with other results later. The in-memory component is just as important. When data is ready and available in-memory, it can be acted upon immediately. Alternatively, data is available on disk (slow storage) and thus any operation or calculation is dependent on the time to load the data into memory. With large data sets, the load time can cause a material impact on the time to make calculations. When near-real-time is the goal, in-memory analytics is the only option.
Industry Perspectives is a content channel at Data Center Knowledge highlighting thought leadership in the data center arena. See our guidelines and submission process for information on participating. View previously published Industry Perspectives in our Knowledge Library.
About the Author(s)
You May Also Like