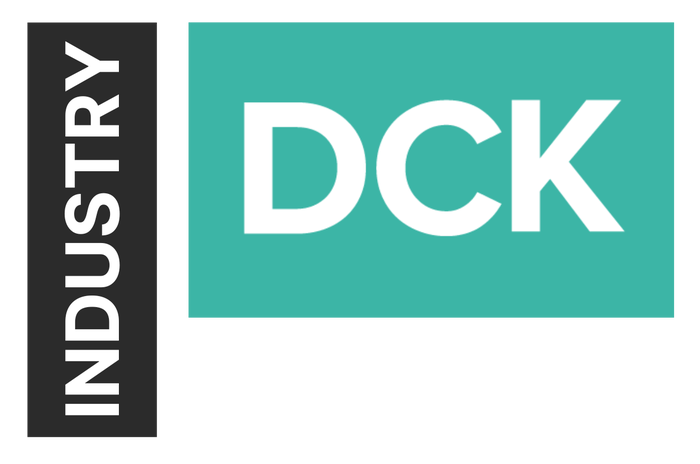
Insight and analysis on the data center space from industry thought leaders.
Bridging the Gap Between the Data Lake and the Data Analyst
Data professionals who wants to future-proof their careers should strive to broaden skillsets beyond traditional statistics, SQL and data visualization.
May 23, 2018
Ori Rafael is the CEO of Upsolver.
The role and attitude toward business analytics has changed dramatically over the past decade. The proliferation of digital data, alongside the rapid growth of a related ecosystem of business intelligence tools - estimated at $18.3 billion by Gartner in 2017 - has created a sea change. Powerful analytics capabilities, previously considered a nice-to-have for large enterprises with deep pockets, have become commonplace.
Today’s companies have become very good at driving insights from structured data in order to improve business performance. However, as companies outgrow the traditional relational database and data warehouse models and gravitate toward streaming data and data lakes, they often hit a brick wall: Data analysts often don’t possess the engineering skills and tools needed to access, prepare and query this data, resulting in a lack of analytical output.
Let’s take a closer look at the gap between modern business analytics and the data lake approach and suggest possible solutions that don’t require a complete overhaul of technology and staff.
The Database, Data Analyst, and Glass Ceiling
The crux of the problem is that modern business analytics was designed around two core concepts: relational databases for storing data, and SQL for querying it. Both of these are ill-suited for the data lake approach.
Relational databases have been used since the late 1970s as a way to organize data in tabular format, according to its relationship with other data, and quickly became standard-issue in the business world. The RDBMS, along with SQL, was revolutionary in the power it gave organizations to answer ad-hoc business questions with data - albeit in a process that today we would view as extremely clunky and IT-intensive, with extensive technical resources required in order to create each new report.
In the late '90s and early 2000s data grew in volume and complexity, but technology was also evolving. Advancements in in-memory processing gave rise to a new generation of analytics tools that could rapidly query a relational database and visualize the results, generating much of the SQL between the scenes. Tasks that were previously relegated to IT teams could now be performed, partially or in-whole, by business units.
This gave rise to a new type of employee: the data analyst, tasked with turning organizational data into business insights. Analysts were well-versed in SQL and relational databases (and later - BI tools), as well as in statistics, data modeling and data visualization - giving them a complete toolset to find useful nuggets of information within raw business data.
As can be seen above, the entire data analytics ecosystem was built around SQL and SQL-based relational databases. This has worked very well and has given almost every enterprise the ability to base its decisions on data and where that data is available. But what happens when the relational database ceases to be the be-all, end-all solution for an organization’s data?
Rise of the Data Lake
In recent years, digital data has taken another evolutionary step in terms of granularity and complexity: If data analysis was previously focused on a handful of systems-of-record (CRM, finance, HR, etc.), many organizations are now setting their sites on streaming data - data that is generated continuously by thousands of sources, typically simultaneously and in small sizes.
This could include, for example, the data generated by sensors in innumerable IoT devices, or digital "events" that track every interaction between masses of users and web applications.
Data-intensive organizations that collect and generate insights from streaming data are replacing or supplementing the traditional database approach with data lakes. These are massive data repositories that collect and store troves of enterprise data in its raw form, which is often unstructured or semi-structured, without forcing the data into a tabular structure or pre-defined schema.
Data lakes pose a major challenge to the current concept of self-service analytics. Data lakes often store unstructured data, which does not lend itself naturally to SQL querying; data is not stored in tabular form; and the size and granularity of the data is often of a completely different scale compared to an RDBMS.
Hence, data analysts find themselves in an awkward position: their skills, and the tools they have grown accustomed to using, no longer suffice to generate the value their organization expects to receive. Data engineers and DevOps are often required merely in order to retrieve the relevant data for analysis, putting analysts in a similar position to where they were with RDBMS 20 years ago.
Bridging the Gap
In light of the above, will organizations be forced to accept that the data analytics technology and knowledge they have amassed are ill-suited for the age of the data lake?
I believe that the answer to this question is negative. Data analysts, with their unique knowledge of the relevant business domains and their ability to translate data into digestible insights, can continue to provide value to organizations within a data lake architecture. However, to enable this, both technology and people need to adopt to the changing times:
On the technology side, there is dire need for simplification. Today’s data lakes are in a similar position to relational databases in the '90s - expensive, complex, and sequestered behind a wall of technical skills that allow only a small subset of highly technical users to actually access the data. In the data lake world, these would typically be big data engineers who are familiar with extremely complicated software packages such as Spark. When every new question an analyst wants to answer has to enter the backlog of an engineering team, the analyst might give up on asking new questions altogether.
However, just as a new category of business analytics tools emerged in order to reduce the complexity of working with databases and connect business units directly to RDBMS data, a similar process is now taking place in the world of streaming data. Modern technology can streamline the process of querying and aggregating streaming data, as well as connecting it to the business analytics tools the organization already uses.
As for the data analysts, they would be well advised to enhance their knowledge of the data lake and its unique intricacies. While the traditional database is probably not going to completely disappear any time soon, streaming data is also here to stay. Data professionals who wants to future-proof their careers should strive to broaden skillsets beyond traditional statistics, SQL and data visualization - delving deeper into coding, predictive analytics and machine learning.
Closing
Data lakes pose new challenges for the business world, forcing organizations to re-evaluate tools and skill sets which were built for a world of relational databases. Data analysts, who have become a staple of today’s data-driven business, are at the forefront of this challenge.
To ensure a seamless transition from databases to data lakes requires a combination of new technology and willingness on the part of data analysts to learn new skills and techniques. Forward-facing organizations should begin to tackle these challenges sooner rather than later.
Opinions expressed in the article above do not necessarily reflect the opinions of Data Center Knowledge and Informa.
Industry Perspectives is a content channel at Data Center Knowledge highlighting thought leadership in the data center arena. See our guidelines and submission process for information on participating.
About the Author(s)
You May Also Like