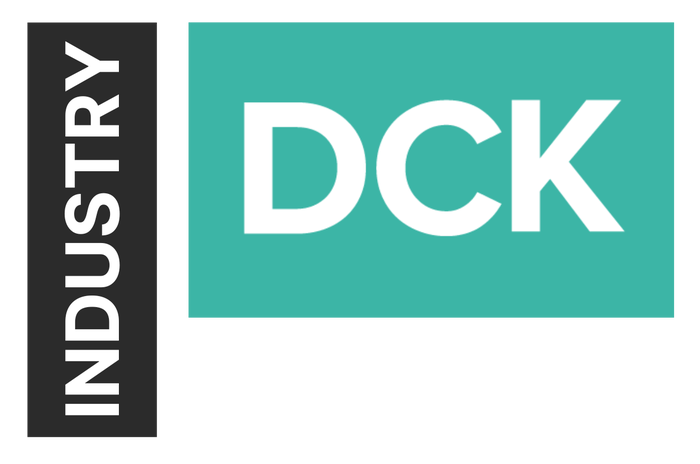
Insight and analysis on the data center space from industry thought leaders.
Five Challenges Companies Must Overcome to Make Use of All Their Data
August 30, 2017
Joe Pasqua is EVP of Products for MarkLogic.
Companies today know they need to fully and effectively leverage all data—including the increasing digitization of human communications and the data being generated by everything from light bulbs to smartphones. They know they must capture a wide variety of data, store it in a way that makes it accessible, and query it based on the rapidly changing needs of the business. They also know that they can’t get by with rigid, predetermined schemas . What they are finding, however, is that this is much easier said than done.
What’s standing in their way? Many things, unfortunately; but there are five big challenges that companies must overcome in order to fully exploit their data along with partner data, and other external data sources.
1. Inability to make use of multiple data types and formats. Data today comes in all shapes, sizes, and forms that must be processed and analyzed basically in real time. This includes data that does not fit neatly into the rows and columns of legacy relational database systems. What’s more, those different forms and types of data need to be used together seamlessly. Richly structured data, graph data, geospatial data, and unstructured data may all figure into a single query or transaction.
2. Slow pace of innovation based on legacy systems. Technology and business requirements are changing almost daily, and organizations need to innovate to stay competitive and compliant. Many companies today can barely deal with the data they have on hand, let alone what will be coming in the future such as IoT-generated data. When investing in innovation, they are often frustrated because they need to deal with legacy systems, which hold many of the corporate data assets. These systems are an anchor that slow their progress and ability to effectively compete.
3. Proliferation of data silos in the enterprise. The rapid growth of all kinds of data and the growth in the number of services businesses provide to their customers, has created a proliferation of data silos in the enterprise. To better serve their customers, regulators, and themselves, businesses need to create a 360-degree view of their business objects such as customers, products, or patients. But creating this holistic view has been an arduous and wildly expensive task. All the while, more data silos are being created. What’s worse, the data quality and the governance of these views is often an afterthought leading to bad results, or even regulatory fines.
4. The use of ETL and schema-first systems. Relational databases are the de facto standard for storing data in most organizations. Once a relational schema is populated, it is simple to query using SQL. Sounds great, but—and this is a big but—companies have to create the schema that queries will be issued against. Integrating all existing schemas (and possibly mainframe data and text content) requires a tremendous amount of time and coordination among business units, subject matter experts and implementers. Then, once a model is finally settled on by various stakeholders, data must be extracted from source systems, transformed to fit the new schema and then loaded into the new schema—a process referred to as ETL. Critical understanding can be lost in all of this translation, and it simply takes too long ( average 6-18 months). Moreover, it never ends. Data sources change. New sources are added. Different questions are posed. ETL keeps on taking, not giving.
5. Lack of context. Perhaps the biggest problem companies have today is thinking they know what they don’t know. Data without context is useless. What does this data mean? How does it relate to other data? What is the provenance of the data? In what circumstances and with whom am I allowed to share it? In most cases the answers to these questions aren’t captured in the database. It might be in a developer’s head, or a design document, or an ETL script, or worse, all those places, but not consistently. Traditional databases aren’t focused on storing, managing, and querying this contextual metadata and typical ETL processes usually drop this information on the floor. Giving up on context means giving up on getting the most value from your data.
So, what’s a company to do? Increasingly, companies are turning to multi-model databases. With a multi-model database, they can capture data’s context and store it with the data, providing maximum data agility and auditability – and essentially future-proofing the database system against any new type of data, shift in data paradigm or regulatory requirement that will inevitably come down the pike.
Companies considering a multi-model database platform should look for:
Native storage of multiple structures (structure-aware)
The ability to load data as-is (no schema required prior to loading data)
Ability to index these different models efficiently
Ability to use all the models together seamlessly – composability
Enterprise-class security and availability
Of course, no shift in database technology is made lightly—many IT professionals have gone their entire careers in one technology. But if there was ever a time for companies to ensure that they can effectively collect, analyze, and leverage the data at their disposal, it’s now.
Opinions expressed in the article above do not necessarily reflect the opinions of Data Center Knowledge and Informa.
Industry Perspectives is a content channel at Data Center Knowledge highlighting thought leadership in the data center arena. See our guidelines and submission process for information on participating. View previously published Industry Perspectives in our Knowledge Library.
About the Author(s)
You May Also Like