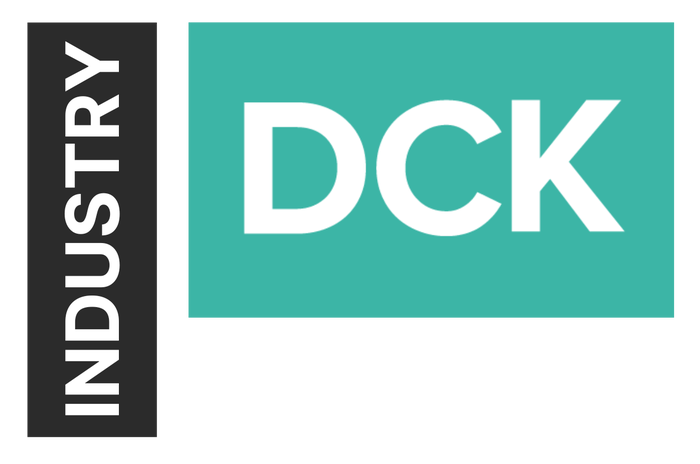
Insight and analysis on the data center space from industry thought leaders.
“Just When You Thought”: How Predictive Analytics Will Impact the Data Center
In the last few years, the value of predictive analytics has become clear, and businesses are clamoring for rapid adoption and deployment, writes Bill Jacobs of Revolution Analytics.
January 12, 2015
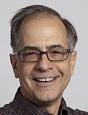
Bill Jacobs is VP of Product Marketing and field CTO for Revolution Analytics.
In the last few years, the value of predictive analytics has become clear, and businesses are clamoring for rapid adoption and deployment.
As pressure mounts to build out analytical frameworks atop the big data “lakes” or “landfills” started in 2013 and 2014, data center teams will be driven to meet a few immediate needs and begin considering some longer-range trends.
Production Analytics: New Platforms
Deployment of predictive analytics to production will deliver operational, performance and risk improvements across large organizations in 2015.
Whether by integrating on-demand analytics into BI dashboards and reports, or by computing predictions as part of the business application logic, predictive analytics will go production for many this year.
For those already in production, historical methods such as re-coding predictive models into Java or C++ prior to deployment have reached end-of-life. As application teams accelerate model deployment cycles, lower development costs and minimize coding inaccuracies, direct deployment of new models is needed.
For IT, this means onboarding of new production systems that compute predictions, and with them, come challenges.
Where transaction or event volumes are high or latency requirements are low, or both, IT will need to deploy in-database, in-Hadoop and streaming analytical components into the application stack. This will keep IT operations, security and business continuity teams busy characterizing and deploying these new components to achieve fast, reliable computation of model predictions.
Sensor Data: Big Volume, Here and Now
The “Internet of Things” or IoT will bring large flows of new data into the data center in 2015. However, while many focus on new types of sensors such as smart thermostats, smart cars, fitness bands and Wi-Fi light bulbs, IT should be wary of the potential impacts of existing data sources as well.
Today, many cars sold in the last five years are already producing data. Most manufacturing machinery also produces large data flows, particularly in the electronics business. Telecom networks have produced large historic data sets as well as large real-time flows of data. Weather and geo-spatial data are widely available, growing rapidly in detail, and can be embraced to great positive effect as modeling techniques and deployment infrastructures make their inclusion a reality.
It is the here and now data that will impact the data center first, providing a training ground for larger and more numerous data flows from new sensor networks this year and next.
Predictive Analytics: A Fit for the Hybrid Cloud?
Much has been written and scores of hosting and platform companies are working toward generalized solutions for creating hybrid clouds that combine public, private and on-prem assets. The reality remains that bandwidth is not free, nor will it be anytime soon.
We predict that in 2015, many organizations will realize that hybrid clouds can prove a good foundation for predictive analytics before generalized solutions are widely adopted.
With the lion’s share of new data originating outside the organization, it makes sense to store and analyze large, new data assets near that origin. However, movement of data between cloud and on-prem remains expensive.
Big data analytics offers a natural division between model development and model execution that fits reasonably well into hybrid cloud realities for many problem sets. By storing large new data assets in cloud-based infrastructure and selecting data modeling tools that can run there, IT can help data modelers simplify and accelerate the ingestion, blending, exploration and model estimation on vast data assets without moving the data.
By pairing in-cloud analysis with on-prem model execution, IT can bridge data modeling directly to production applications without re-engineering mission critical apps to run in the cloud, and without moving massive data sets between the cloud and on-prem infrastructures.
The future will reveal new architectures for hybrid clouds and analytics, modeling atop data lakes in the cloud, and deploying those models on-prem. This matches the realities of today’s hybrid clouds and provides a great starting point. Evolution of hybrid cloud architectures will smooth seams visible in today’s hybrid cloud architectures. As hybrid cloud facilities mature, they will also pave the way for innovative analytical techniques like online learning algorithms that blur the current distinction between model development and model execution.
Deploying predictive analytics to production, embracing big data from sensor-based networks, and fitting production analytics to the realities of hybrid clouds are but a few of the interesting challenges to be met in 2015. I welcome your comments on what you envision as the most import barriers to your company or organization. Good luck in 2015!
Industry Perspectives is a content channel at Data Center Knowledge highlighting thought leadership in the data center arena. See our guidelines and submission process for information on participating. View previously published Industry Perspectives in our Knowledge Library.
About the Author(s)
You May Also Like