NIST Creates Cybersecurity Playbook for Generative AI
NIST identifies various attacks AIs could face and proposes ways to mitigate them.
January 8, 2024
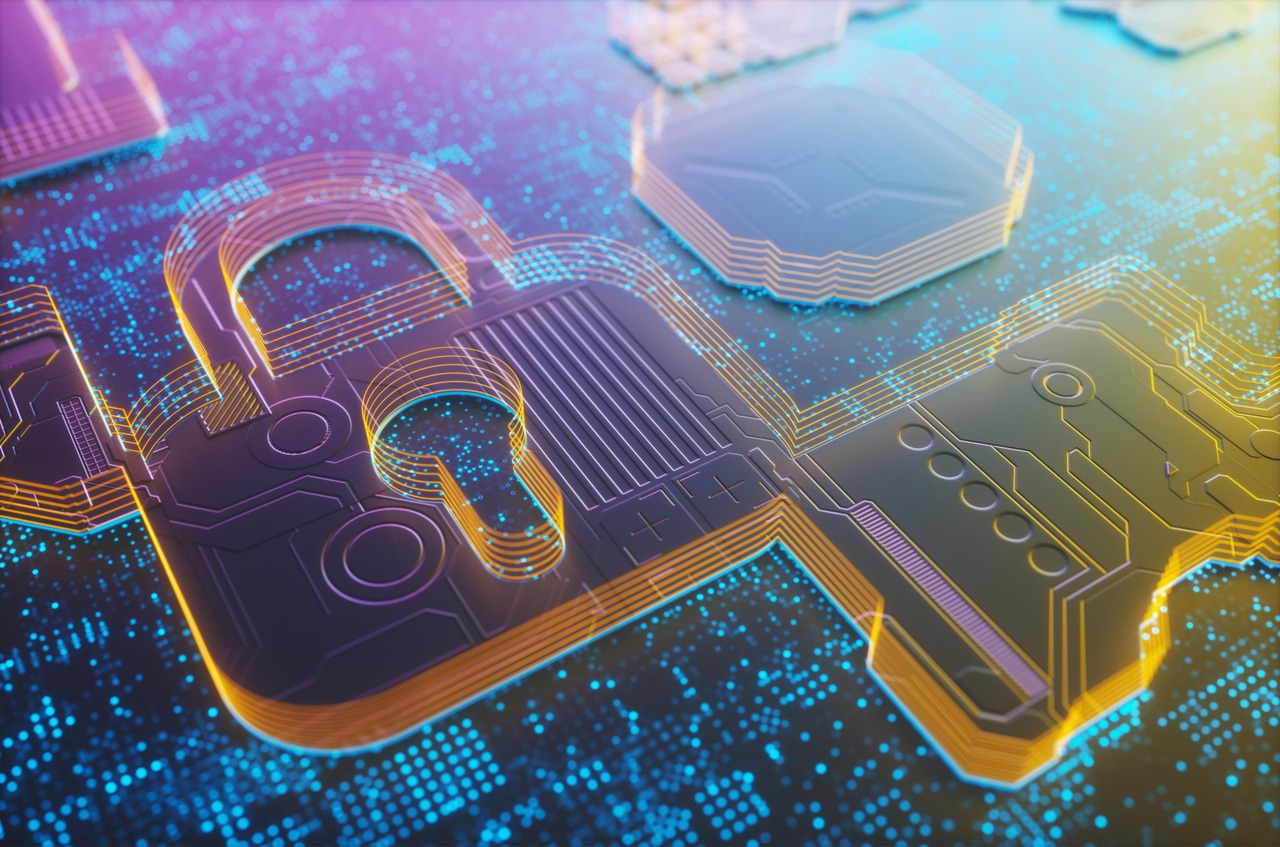
The US National Institute of Standards and Technology (NIST) has published a report laying out in detail the types of cyberattacks that could be aimed at AI systems as well as possible defenses against them.
The agency believes such a report is critical because current defenses against cyberattacks on AI systems are lackluster – at a time when AI is increasingly pervading all aspects of life and business.
Called “Adversarial Machine Learning: A Taxonomy and Terminology of Attacks and Mitigations,” the report starts by developing a taxonomy and terminology of adversarial ML, which in turn will help secure AI systems as developers have a uniform basis from which to form defenses.
The report covers two broad types of AI: predictive AI and generative AI. These systems are trained on vast amounts of data, which bad actors may act to corrupt. This is not inconceivable since these datasets are too large for people to monitor and filter.
NIST wants the report to help developers understand the types of attacks they might expect along with approaches to mitigate them, though it acknowledges that there is no silver bullet for beating the bad guys.
NIST’s identifies four major types of attacks on AI systems:
Evasion attacks: These occur after an AI system is deployed, where a user attempts to alter an input to change how the system responds to it. Examples include tampering with road signs to mess with autonomous vehicles.
Poisoning attacks: These occur in the training phase through the introduction of corrupted data. Examples include adding various instances of inappropriate language into conversation records so a chatbot would view them as common use.
Privacy attacks: These occur during deployment and they are attempts to learn sensitive information about the AI or the data it was trained on with the goal of misusing it. A bad actor would ask the bot questions and use those answers to reverse engineer the model to find its weak spots.
Abuse attacks: These involve inputting false information into a source from which an AI learns. Different from poisoning attacks, abuse attacks give the AI incorrect information from a legitimate but compromised source to repurpose the AI.
However, each of these types can be impacted by criteria like the attacker’s goals and objectives, capabilities and knowledge.
“Most of these attacks are fairly easy to mount and require minimum knowledge of the AI system and limited adversarial capabilities,” said Alina Oprea, co-author and a professor at Northeastern University. “Poisoning attacks, for example, can be mounted by controlling a few dozen training samples, which would be a very small percentage of the entire training set.”
Defensive measures to mount include augmenting the training data with adversarial examples during training using correct labels, monitoring standard performance metrics of ML models for large degradation in classifier metrics, using data sanitization techniques, and other methods.
About the Author(s)
You May Also Like