IBM’s Machine Learning Tech Takes on Solar Power’s Flakiness
New modeling and forecasting system said to improve forecast accuracy by 30 percent
July 22, 2015
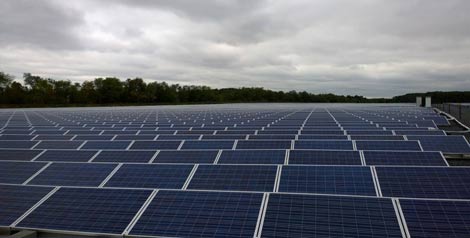
By Joanna Glasner
Some of the biggest data center operators, companies like Google, Facebook, and Microsoft, buy a lot of renewable energy to clean up the power supply of their cloud infrastructure. Yet, their ability to rely on wind and solar has long been limited by changing weather patterns and their effect on generation levels, putting renewables in the category of “intermittent” energy sources.
Now, through the power of Big Data and machine learning, new forecasting technology promises to alleviate some of that uncertainty.
Earlier this month, IBM disclosed that solar and wind forecasts it co-developed using machine learning technologies are proving to be as much as 30 percent more accurate than ones created using conventional approaches. Called the Self-learning weather Model and renewable forecasting Technology, or SMT, it continuously analyzes and improves solar forecasts derived from a large number of weather models.
Forecast accuracy is better primarily thanks to the sheer enormity of the datasets available, Hendrik Hamann, manager of the Physical Analytics group at IBM Research, said. He is part a team of more of than a dozen in-house researchers, working in collaboration with the Department of Energy, who spent over two years developing the forecasting technology.
The project used up a big chunk of the petabyte of storage dedicated to it, tapping into the DoE’s high-performance computing facilities for processing power. Data sources include sensor networks, local weather stations, cloud motion tracked by sky cameras and satellites, and historical records going back several decades. Variables are plugged into multiple forecasting models, with the system continuously tracking how they work under varying conditions.
“We can actually see which one of those models or forecasting systems has performed better than others,” said Hamann, adding that the technology’s applications aren’t confined to solar. Researchers are looking to apply similar machine learning systems for wind and hydro power, and Hamann sees potential for application in other industries, such as tracking soil moisture levels for agriculture.
Over the next year, the focus will be on integrating the solar forecasting technology into the operations of energy producers. IBM and the DoE are working with operators including ISO New England, an electricity provider for six New England states, on putting the system to practical use. Implementation is particularly timely for the New England region, which in the last five years has gone from just 44 MW of installed solar photovoltaic resources to 1,000 MW.
Meanwhile, power companies in virtually all geographies are adding solar capacity at a brisk pace. In 2013, solar was the second-largest source of new electricity generating capacity in the US, exceeded only by natural gas. However, the difficulty in producing accurate solar and wind forecasts has required electric utilities to hold higher amounts of energy reserves as compared to conventional energy sources.
A key goal for the DoE is to make it easier and more cost-effective to implement solar. Funding for the forecasting project came from the department’s SunShot Initiative, a federal research program aimed at making solar fully cost-competitive with traditional energy sources before the end of this decade. If progress is on track, SunShot researchers predict that solar power could provide as much as 14 percent of US electricity demand by 2030 and 27 percent by 2050.
The team of scientists from IBM and the National Renewable Energy Laboratory are presenting a paper on their preliminary findings this week at the European Control Conference in Linz, Austria.
About the Author(s)
You May Also Like