4 Ways to Optimize Your Data Center for AI Workloads
To enhance your data center's capabilities for handling AI workloads, consider making these changes that address the unique needs of AI.
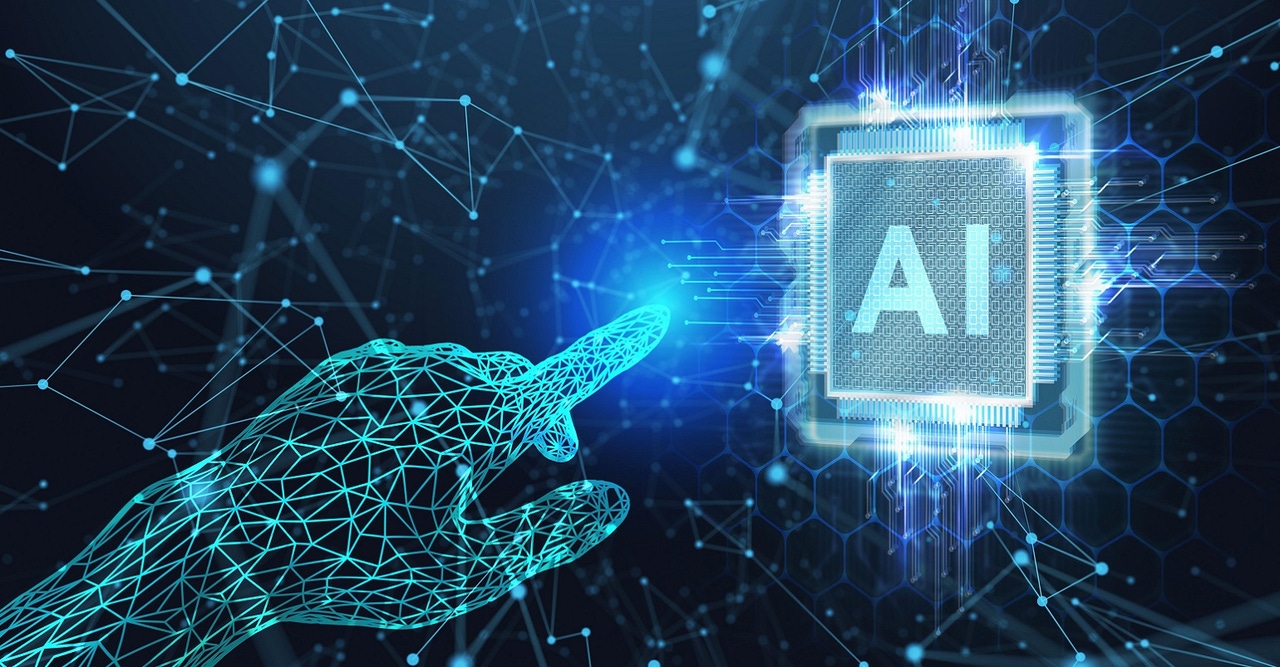
AI is poised to transform data centers in many ways — such as by changing the data center job market and improving data center monitoring and incident response operations.
Yet, perhaps the greatest impact that AI is likely to exert on data centers will come in the form of changes to the way data centers work. The infrastructure that facilities house and the way they manage it must change for businesses that want to take full advantage of modern AI technology.
Exactly how data centers will evolve in response to AI remains to be seen, but here's a look at several key changes to expect.
The Unique Needs of AI in Data Centers
To assess the impact of AI on data centers, you must first understand how AI workloads differ from other types of workloads (such as standard application hosting) that you'd encounter in a data center.
While AI workloads come in many forms with varying requirements, most are subject to the following unique needs:
They require massive amounts of compute resources, especially when performing model training.
They benefit from running on bare-metal hardware, particularly servers that provide access to graphical processing units (GPUs).
Their resource consumption rates may fluctuate significantly. During the training phase, AI workloads require tremendous resources, but after training is complete, resource consumption goes down significantly in most cases — until it's time to retrain the model.
They need ultra low-latency networks in order to make decisions and deliver results in real time.
To be sure, other types of workloads may also have these requirements; running AI apps and services is not the only type of use case that can benefit from bare-metal servers, for example. But by and large, AI software requires the types of resources described above to a much greater degree than other types of workloads.
Updating Data Centers for AI
To optimize their facilities for AI workloads, many data center operators will need to make changes that address the unique needs of AI. Here's a look at key data center updates on this front.
1. Redesigning or replacing bare-metal servers
For at least the past decade, virtual machines have been the go-to infrastructure resource for hosting workloads. But given the need of AI apps and services for bare-metal hardware, more data center operators will likely find it important to expand bare-metal offerings.
In some ways, this actually simplifies data center operations. If you run workloads on bare metal, you end up with a less complicated hosting stack because you don't have hypervisors and VM orchestrators in the mix.
On the other hand, expanding bare-metal infrastructure for hosting workloads may require data centers to update the types of servers they host and the racks in which the servers live. Conventionally, the simplest way to set up servers in a data center was to acquire very powerful bare-metal machines, then divvy them up into as many VMs as your workloads required. But if you need to run workloads directly on bare metal, you may need more servers in order to isolate workloads — which would mean that data centers would have to swap out high-power servers for smaller ones, and possibly update server racks accordingly.
2. Shared access to GPU-enabled servers
Although many AI workloads can benefit from GPU-enabled servers when performing training, AI apps don't necessarily need GPUs for everyday operations. For that reason, many businesses only require access to GPU-enabled infrastructure on a temporary basis.
To meet that demand, data center operators should consider offerings that allow companies to share access to GPU-enabled infrastructure. Relatively few businesses are likely to want to own GPU-equipped servers because they won't need them on a permanent basis. But if data center operators can provide access to GPUs on a temporary basis — such as via a GPU-as-a-service model — they are in a stronger position to attract businesses with AI workload requirements.
3. Enhanced networking solutions
Most enterprise-grade data centers already provide access to high-performance network infrastructure, as well as interconnects that help move data to external facilities as rapidly as possible. But to take full advantage of AI, data center networking offerings will likely need to become even more robust.
Businesses with AI workloads will be looking for two key features: first, high-bandwidth network connections that can move massive amounts of data very quickly, which is important when training AI models on distributed infrastructure. And second, they'll want networks that can deliver single-digit latency, which is essential if you want AI apps and services to perform in true real time.
4. More data center flexibility
Because AI workloads have widely fluctuating resource requirements, they will probably create demand for data centers that are more flexible in terms of how much infrastructure they need to support. AI may also drive more interest in services that allow companies to deploy servers on demand inside someone else's data center, rather than setting up those servers themselves, since on-demand infrastructure is a good way to address fluctuating resource needs.
To this end, data center operators who want to optimize for AI should consider offerings that make their facilities more flexible. Shorter-term contracts, combined with services that include more than just rack space where customers can set up their own infrastructure, are likely to help attract organizations that need to deploy AI workloads.
Conclusion
Again, the AI revolution is still playing out, and it's too soon to know exactly how AI will transform the way data centers operate or the type of infrastructure deployed in them. But it's a relatively safe bet that changes like more GPU-enabled servers and more flexible solutions are likely to prove critical in an AI-centric world. Data center operators who want to capture their piece of the AI pie should be sure to update their facilities in ways that cater to the special requirements of AI workloads.
About the Author(s)
You May Also Like