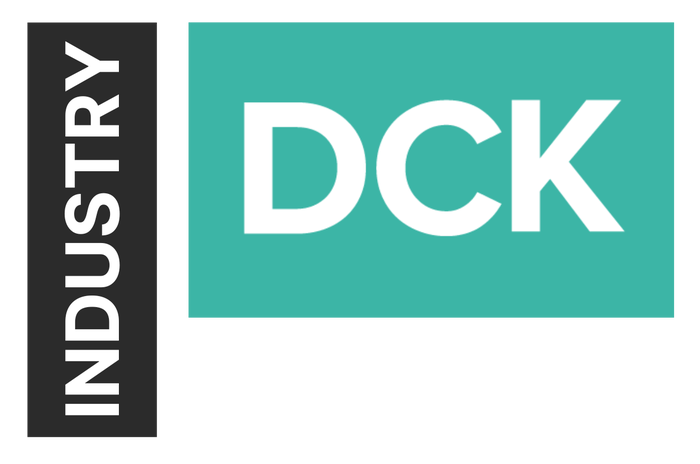
Insight and analysis on the data center space from industry thought leaders.
Self-Service Analytics are Not Sustainable
Business users don’t need access to more raw information; they need tools that take the leg work out of data analysis and automatically surface them the insights they need so they can focus on doing their jobs better.
December 9, 2017
Roman Stanek is CEO at GoodData.
In the last five years, Business Intelligence (BI), data and analytics vendors have all focused on delivering tools that are "self-service," granting a typical business user the ability to approach data and analytics without a background in statistical analytics, BI, or data mining. These self-service solutions turned legacy BI on its head by offering a powerful promise: Users of all skill levels would be able to operate complex software with a few easy clicks to make radically better business decisions, freeing analysts and data scientists to focus on strategic work rather than reporting. This sounds Utopian, but there’s a problem: It’s not sustainable.
On the surface, data democratization sounds ideal. The amount of raw data that business of all types generate as part of their daily operations has grown exponentially, so the idea of giving everyone in the organization (not just the “Data Elite”) the ability to derive insights and value from this information is extremely attractive. But as the flood of data continues to increase, the ability of these tools to provide clear insights is rapidly degrading. And simply trying to cram more information into these tools will only compound the problem; as Emperor Joseph told Mozart, “there are only so many notes an ear can hear over the course of an evening.”
"By 2018 most business users will have access to self-service tools, but the fact remains that there’s too much data for the average business user to know where to start." said Anne Moxie of Nucleus Research. “What we are starting to see is a new generation of BI where mundane daily tasks are automated while flagging more significant anomalies for employees to focus on.”
The fundamental problem is that "self-service" tools require business users to spend more and more of their time digging through data, but an average employee can’t be expected to reliably self-identify the intricate patterns in that data that could be either an important early warning sign, or nothing at all. More importantly, they should not have to. Business users don’t need access to more raw information; they need tools that take the leg work out of data analysis and automatically surface them the insights they need so they can focus on doing their jobs better.
The solution to this problem lies in harnessing advances in machine learning and artificial intelligence to automate analysis and surface actionable insights where they’re needed most: at the point of work. Embedding these “Smart Business Applications” into the tools that business users are already using every day will increase the penetration of data and impact of these recommendations, without every employee in the organization needing hours of training to master BI tools.
Imagine how powerful it would be if, rather than having to use a separate BI platform to self-perform complex data analysis, your employees were presented with simple, reliable and machine-accurate recommendations for the next business actions they should take directly inside the core business applications they use every day. If implemented correctly, this combination of embedding and machine-learning capabilities can deliver in-context automation, recommendations and insights that can truly deliver tangible results. These Smart Business Applications can automate many of the low-level decisions that business users face, freeing them up to focus on more strategic and impactful problems. The applications are limitless, but could be game changing for industries like:
Financial Services: Loan officers need automated insights at scale and in real-time to make the most risk-averse decisions possible. While algorithms are already being used to recommend approval or denial of loans, these are antiquated systems. Using machine learning, these systems can be augmented and enriched to include unstructured data from social media, IoT, buying behavior and more to paint a much more accurate picture of applicants far more quickly.
Retail: Scorecarding and benchmarking is not enough. Adding predictive analytics around expected customer segmentation and product demands and combining them with real-time data about how products are selling will give product manufacturers a better view of how they are performing and what they should change in the future.
Healthcare: Machine learning will allow brands to access data from across their entire provider network to benchmark hospital/doctor performance against industry regulated KPIs and improve operating margins.
Accounting: Advances in predictive analytics will allow accounts payable to apply lessons learned from hundreds of thousands of transactions to process invoices more efficiently and identify fraudulent claims automatically to flag investigations.
With the current generation of self-service BI tools, we’re asking everyone in the organization to become data scientists and business experts. And from everything I’ve seen, that’s not reasonable, and it’s not happening. Organizations should offload the work of analyzing reports to a trusted analytics partner and focus on giving their people the tools they need to get back to work. Machines are getting better at extracting insights from complex data than humans are, and companies need to invest in BI solutions that take advantage of advances in machine learning, predictive analytics and artificial intelligence to truly deliver data in-context with clear insights and machine-accurate recommendations.
Opinions expressed in the article above do not necessarily reflect the opinions of Data Center Knowledge and Penton.
Industry Perspectives is a content channel at Data Center Knowledge highlighting thought leadership in the data center arena. See our guidelines and submission process for information on participating. View previously published Industry Perspectives in our Knowledge Library.
About the Author(s)
You May Also Like